How to Write a Systematic Review Civil Engineering
- Review
- Open Access
- Published:
Value of information analysis in civil and infrastructure engineering: a review
Journal of Infrastructure Preservation and Resilience volume 2, Article number:16 (2021) Cite this article
-
1789 Accesses
-
2 Citations
-
1 Altmetric
-
Metrics details
Abstract
The concept of Value of Information (VoI) has attracted significant attentions within the civil engineering community over especially the last decade. Triggered by the increasing focus on structural health monitoring, availability of data and emerging techniques of Big Data analysis and Artificial Intelligence, important insights on how to take benefit from VoI in structural integrity management have been gained. This literature review starts out with a summary of the historical developments and contains (1) a summary of two different VoI analysis origins, (2) a compilation of existing VoI analyses research and (3) current engineering interpretations and applications of VoI in the field of civil and infrastructure engineering. VoI analysis has roots in communication theory and Bayesian decision analysis in conjunction with utility theory. Starting point is thus taken in brief introduction of these theoretical foundations, followed by a discussion on the relevant modelling aspects such as information, probability and utility modelling. A detailed review of relevant existing research is presented, divided into the following main areas: computational methods, optimal sensor placement and engineering risk management. Finally, by way of conclusion and outlook, challenges and some promising directions for VoI analysis in the field of civil and infrastructure engineering are identified.
Introduction
Decisions in the context of civil and infrastructure engineering are generally subjected to significant uncertainties. This concerns e.g. our understanding of structural degradation, extreme load events, effects of interventions, and quality of workmanship. These uncertainties in turn imply that we do not know with certainty what effect different decision alternatives will have and this poses a rather significant challenge for decision makers. As a means for managing and reducing some of the major uncertainties decision makers increasingly take benefit from the possibility to observe the performances of building structures and infrastructures more generally through various technological means such as: Non-destructive Testing (NDT), Proof Load Testing (PLT), Structural Health Monitoring (SHM) and Remote Sensing (RS). The information collected thus informs decisions with information on actual performance and thus helps reducing the impact of epistemic uncertainty on the uncertainty associated with the different available decision alternatives. Over the last half century, the application of these technological tools in civil and infrastructure engineering has yielded a number of results [1–4], which have contributed importantly to ensuring and documenting the safety of engineering structures, reducing the probability of accidental structural failure and reduced the life cycle costs associated with engineering structures. However, a question arises, that is, how to deal with the broad range of sometimes very significant amounts of data associated with structural performance? In the field of data science and information management, the Data-Information-Knowledge-Wisdom (DIKW) model has been proposed to represent the structural and/or functional relationships between data, information, knowledge and wisdom in order to support decision making. Similarly, in engineering practice, a DIKW model can be utilised to frame the context of decision making for integrity management, see Fig. 1 [5]. As shown in Fig. 1, the information collected can enhance the quality of engineering risk management. Since the aim of decision-making is to rank decision alternatives relative to their expected utility, it is only natural that additional information, which can be obtained from the available data, should be utilised. The concept of Value of Information (VoI) provides the theoretical foundation for quantifying the potential benefits of additional information, derived from Bayesian decision theory, which has gained much attentions in recent years and is widely used in the field of civil and infrastructure engineering. A useful way of describing VoI is to contrast an act-then-learn approach with a learn-then-act approach [6]. Based on the concept of VoI, decision makers can quantify the potential benefits of additional information and thus determine whether corresponding campaigns of collecting information, e.g. through measurements, should be undertaken.
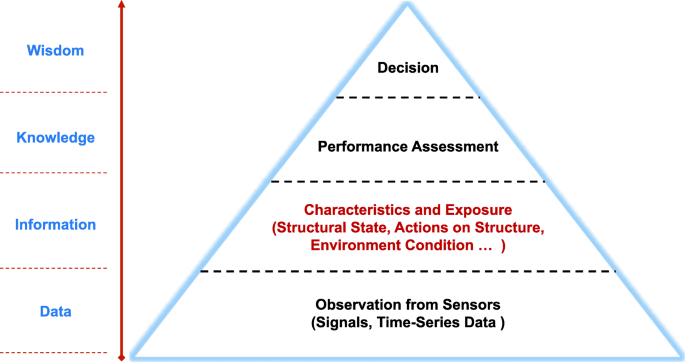
The DIKW structure for the engineering decisions [5]
Full size image
The present paper presents a literature review of existing research applications of VoI in the field of risk analysis and decision making in civil and infrastructure engineering, with the hope of providing researchers with a systematic review to aid future research in this field. This work starts out with a brief outline of the history of VoI developments, followed by an axiomatic description and classification of VoI definitions and analysis methods. Next, the considerations of various modelling aspects in the VoI analysis are categorised and reviewed, subsequently, the present work gives a summary of existing studies and investigations in the field of civil and infrastructure engineering. Finally, the summary and promising prospects of VoI analysis are presented and discussed.
A brief history of value of information analysis
The quantification of VoI has during 1950ies and 1960ies originated from two different fields of science, namely (1) information and communication theory, and (2) Bayesian decision theory. In the domain of information and communication theory, VoI has been derived by assigning utilities to probabilistically defined states and by quantifying the induced values associated with the uncertainty reduction of information. On the contrary, Bayesian decision theoretic definition takes basis in assigning probabilities as weights to a cost-benefit optimisation and then defining the difference to an expected utility optimisation without information as the value of information. Both definitions are utilized in the reviewed literature, however, in the field of engineering risk analysis, Bayesian decision theory by far and large dominates.
An early approach of utilizing information for uncertainty reduction can be found in Communication Theory [7], quantifying the contribution of additional information to the reduction of uncertainty in the system of relevance through combining the Shannon's Information Entropy with Statistical Decision Theory. Hereafter, in Mccarthy [8], it was found that any convex function of a set of probabilities may serve as a measure of VoI. Furthermore, as stated in Howard [9], "no theory that involves just the probabilities of outcomes without considering their consequence could possibly be adequate in describing the importance of uncertainty to a decision maker", so the utility associated with the corresponding action and uncertainty should be taken into account when measuring VoI. A Bayesian formulation of VoI was introduced in Raiffa and Schlaifer [10] based on the Expected Utility Theory (EUT) [11] around 1960 through the pre-posterior decision analysis. They firstly define the VoI in the context of business decisions in their classical textbook, which provides the first axiomatic definition of VoI in decision theory together with the consideration of the uncertainty associated with or contained in the information. On the basis of existing research, a more in-depth discussion on the VoI is carried out in Feltham [12], in which the information attributes of relevance and delay in decision-making scenarios and the perception of decision-makers are incorporated into the framework of the VoI analysis. Miller [13] noted the problem in the value of sequential information analysis, and found through analysis of arithmetic examples that when there is a range of information, the order in which the information is obtained has a significant impact on VoI, so that making decisions in a sequential decision situation is best done through sequential analysis. In summary, at the early stage, it can be seen that the study of VoI in both information theory and economics essentially return to the fundamental point of the contribution of information to the reduction of the expected value of losses (or increase in expected value of gains), and both utilize VoI to inform decision makers in choosing the utility maximising action before implementing it, to reduce the epistemic uncertainties affecting the decision problems. Since then, Bayesian decision analysis and VoI analysis have attracted substantial interests and been applied in a broad range of research and application fields including economics, informatics, medical, and environmental risk management [6, 14–16]. In terms of the analysis method, pre-posterior decision analysis is the most fundamental method, the details of relevance will be illustrated in the following section.
In the field of civil and infrastructure engineering, the significance of Bayesian decision analysis as a means for engineering optimization has been appreciated already since the 1970s [17, 18]. Hereafter, the concept of VoI has been applied with success especially in the context of optimal planning of operation and maintenance [19, 20]. The combination of Markov decision process (MDP) and VoI for developing the optimal maintenance and rehabilitation decision on the infrastructure management is investigated in [21], in which the VoI is utilized to evaluate the potential benefits of different measurement technologies with the cost-effectiveness consideration. The utilization of the concept of VoI as a means in risk-based inspection (RBI) is investigated in [22] as a means to optimize decisions on where and how to inspect, when and how to repair. With the emerging of SHM systems, VoI analysis has aroused high interests in the context of decision making with respect to SHM system arrangement and operation [23–25]. Since then, not least through the COST Action TU1402 [26], substantial further developments and applications of VoI analysis in the context of SHM have been undertaken. The methodologies and research frameworks on how to apply the VoI analysis of SHM systems, how to analyze the VoI of SHM systems and how to optimize the arrangement strategy of SHM systems have attained extensive attentions and been subjected to numerous investigations [27–30]. The application of VoI analysis in the field of civil and infrastructure engineering is reviewed in detail below. As a means for clarifying the VoI concept and associated relevant computation methods, in the following two different perspectives with respect to the concept of VoI will be illustrated and discussed.
Two different perspectives on the value of information
As mentioned above, at the early stage, the concept of VoI has two different interpretations from two distinct application fields. In information theory value is associated directly with entropy, which is more associated with the theory of experiment planning from statistical estimation, where experiments are optimized such as to reduce an invariant of the covariance matrix (of parameters to be estimated), see e.g. [31, 32]. Whereas in Bayesian decision analysis, value is associated with the expected value of utility or consequences, which is more in line with the economic and management perspective. Therefore, this section will be partitioned into two categories to illustrate: (1) Information theory perspective, which deals only with uncertainty in the VoI analysis, and (2) Bayesian decision theory perspective, which considers not only uncertainty but also consequences.
Information & communication theory perspective
The VoI theory is an independent branch of information theory, but is rooted in communication theory [33]. In the field of information and communication theory, the concept of VoI connects Shannon information theory with statistical decision theory, which can be described as the maximum benefit that can be gained in the process of minimizing the expected cost from a given amount of information. The main focus in the area of information theory is the inherent uncertainty in a random variable, which Shannon's entropy can appropriately measure [7]. On the basis of entropy, suppose ξ is the hypothetical variable and entropy is used as the value function, then the value function, V(ξ), is defined as:
$$ V(\xi) = {H(\xi)} = - \sum\limits_{\xi} {P(\xi)\ln P(\xi)} $$
(1)
where ξ is a random variable, for illustration, ξ is assumed as a discrete random variable, and thus P(ξ) is its probability.
Further, suppose ε is a random variable, which can be observed by experiments or tests, then the value function of ξ given an observation on ε can be written as:
$$ V(\xi|\varepsilon) = H(\xi|\varepsilon) = H(\xi,\varepsilon) - H(\varepsilon) $$
(2)
Therefore, the VoI of ε can be expressed as the difference between the entropy for observing and not observing ε:
$$ {\text{VoI}}(\varepsilon) = V(\xi) - V(\xi|\varepsilon) = I(\xi,\varepsilon) $$
(3)
where I(ξ,ε) is the mutual information of ξ and ε, which is in principle a measure of the distance between P(ξ,ε) and P(ξ)P(ε). More details on the assessment of VoI based on the concept of information entropy can be found in [33, 34]. Originating from the viewpoint of reduction of the uncertainty, some studies extended the metric of VoI by establishing novel utility functions considering the entropy-related concepts, like Kullback-Leibler (K-L) Divergence, in the field of civil and infrastructure engineering associated with the sensor optimum arrangement strategy, which will be introduced later.
Bayesian decision analysis perspective
In the field of economics and management, the focus is on how to make optimal decisions under uncertainty, which is closely related to the utility function. As stated in Raiffa and Schlaifer [10], the increase in utility that results from learning additional information and therefore altered prior optimum decision a pri will be regraded as VoI. In the following, the original definition of the VoI analysis in Raiffa and Schlaifer [10] will firstly be demonstrated, which is illustrated through the form of decision tree. Then an analytical form in MDP will be presented, which is an efficient method for the special case that the underlying process (to be controlled) follows a random process with Markovian properties [35].
Representation in decision tree
The decision tree is used as a visual and analytical decision support tool to calculate the expected utility of competing alternatives, which is simple to understand and easy to interpret and generally consists of three types of nodes: decision nodes, chance nodes and utility nodes [36]. For illustrating the concept of VoI, a simple decision tree in [37] is shown in Fig. 2, which represents the pre-posterior decision analysis scenario.
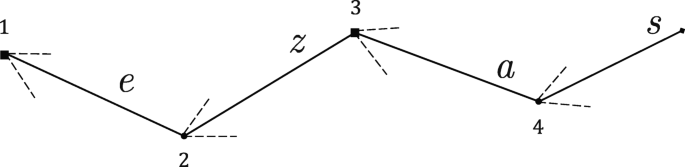
Illustration of preposterior decision analysis in decision tree [37]
Full size image
Let u(e,z,a,s) denote the utility of performing a particular experiment e, observing a particular outcome z, and choosing a particular terminal act a, when the true state of the world is s. It can be seen that u(e,z,a,s) could be decomposed in:
$$ u(e,z,a,s) = {u_{t}}(a,s) + {u_{s}}(e,z) $$
(4)
where u t (a,s) is the terminal utility that results from taking action a when the true state is s, u s (e,z) is sampling utility incurred by performing the experiment e which results in the outcome z.
For any experiment or sample e, the VoI can be defined as the difference of utility between the scenarios of given and not given information, for echo the illustration in the next section, the VoI for this perspective will be called the expected value of information (EVI), which can be formulated as:
$$ {\mathrm{EVI(}}e{\mathrm{)}} = {E_{Z}}[{u_{t}}({a_{z}},z)] - {u_{t}}({a_{0}}) $$
(5)
where a z is optimal action depending on the particular outcome z from the given experiment e, a 0 is the optimal action without additional experiment e. The next section will show that EVI will be positive only if the action a is changed, but if the action a is the same with and without the information given, EVI will be zero. The EVI is the gross value of information, in which the sampling utility u s (e,z) is not considered. To get the net gain, the expected net gain of sampling, ENGS, is defined as:
$$ {\mathrm{ENGS(}}e{\mathrm{)}} = {\text{EVI}}(e) + {u_{s}}(e) $$
(6)
where u s (e) is the sampling utility, which is normally defined as a form of the cost function with a negative value of utility.
However, the full decision tree is computationally intensive in a sequential decision scenario over the service life, therefore, to reduce the computation cost, some simplified methods should be considered [38]. In the following, MDP-based definition will be given in detail.
Representation in Markov decision process
MDP is beneficial when the decision problem involves risks that persist over time and the dynamic environment can be modelled as a Markov process, the details of MDP and the comparison between decision tree could be found in [39].
A MDP [40] constitutes an extension of a Markov chain [41] by introducing actions and rewards associated to states. MDP is mainly used to model and solve dynamic decision analysis with stochastic properties satisfying the Markov property of past state independence, the most basic type of a MDP is the discrete time MDP, which is thus defined with a vector:
$$ \left[ {S,A,{P_{t,t+1}}(a),R(s_{t},s_{t+1},\pi(s_{t})),V} \right] $$
(7)
where S is the state space, which can have a system dimension (as an allocation of system components) and a temporal dimension; when the state is s t ∈ S at time t, an action a from the action space A should be made following a policy π(s t ); P ss+1(a) is the transition probability of the system will transfer from state s t to state s t+1 and R(s t ,s t+1,π(s t )) is the corresponding reward received by the state s t transfer to the state s t+1 under policy π(s t ); V is the objective function, which may be different for different horizon of time [42]. For a finite horizon with time period T, an optimal policy π ∗ (s),s=[s 1,s 2,...,s t ]T, can be obtained by Eq. 8, which is written in the form of the discounted expected total reward:
$$ \begin{aligned} {\pi^{\ast}}(\mathbf{s}) &= \mathop {\arg \max }\limits_{\pi^{\ast}(\mathbf{s}) \in \Pi(\mathbf{s})} {V} \\&\quad= \mathop {\arg \max }\limits_{\pi^{\ast}(\mathbf{s}) \in \Pi(\mathbf{s})} E_{S}\left[ {\sum\limits_{t = 1}^{T} {{d^{t}} \cdot R\left({{s_{t}},{s_{t+1}},\pi(s_{t})} \right)}} \right] \end{aligned} $$
(8)
where the d is the discount factor which has the function of calculating the reward at the time t, Π(s) is a set of possible policies. As such, the objective function with the optimal policy can be written as:
$$ {V^{\mathrm{*}}}{\mathrm{(}}\mathbf{s}{\mathrm{) = }}V({\pi^{\ast}}(\mathbf{s})) $$
(9)
Further, a partially observable Markov decision process (POMDP) is an extension for partial observability, i.e. when the state is not known at the time of the action as introduced by Åström [43], which is a more suitable model that can be employed in engineering risk management due to the limitation of MDP [44]. Additionally to the definitions of the MDP above, the following definitions are introduced: observation space, Ω, and conditional observation probability, O(o k |s t+1,π(s t )), where o k ∈ Ω. The objective function can be written as the MDP objective function, however, the policy function is reformulated in dependency of the observations and their probabilities. It should be noted that the planning with POMDP leads to the algorithm dependency of the solution, i.e. that there is no general algorithm, which can uniquely solve a POMDP. However, there are subclasses of decidable problems, see e.g. [45].
On the basis of the above illustration, the VoI with Markov processes can be defined as the optimal reward difference with and without additional information and their optimal policies (see e.g. [46–51]):
$$ {\mathrm{VoI(}}\Omega{\mathrm{) = }}{E_{\Omega}}\left[ {{V^{\ast}}({\mathbf{s^{\prime}}}|\mathbf{o})} \right] - {V^{\ast}}(\mathbf{s}) $$
(10)
where \({{\mathbf {s^{\prime }}} = [s^{\prime }_{1},s^{\prime }_{2},...,s^{\prime }_{t}]^{\mathrm {T}}}\) is the set of the updated probabilistic models of the state space based on the observations.
Basic procedure for value of information analysis
As stated in the foregoing, there are two different methods for assessing VoI: decision tree analysis and MDP. Although the basic assumptions here are fundamentally different, the procedures and basic ideas of the VoI analysis are much the same, the description of the VoI analysis will then be presented as a decision tree in accordance with the Raiffa and Schlaifer [10].
The essential issue in engineering decision-making is to identify the decision scenario and establish the corresponding feasible representation, through a decision/event tree. This step should follow the hazard identification and uncertainty modelling as outlined in [52]. On the basis of a feasible representation of the relevant decision scenarios, including the decision alternatives, the corresponding probabilistic models, together with the proper assignment of utility, the numerical evaluation of the risks associated with the individual decision alternatives may be performed. Depending on the state of information at the time of the decision, three different analysis types are distinguished: prior analysis, posterior analysis and pre-posterior analysis [53].
The prior decision analysis can be regarded as "decisions with given information", the representation of the prior decision scenario is illustrated by decision tree in Fig. 3. Assuming that the collection of structural state is represented by the random vector Θ with the prior probability distribution \({{\mathop {f}\nolimits }_{\mathbf {\Theta }} }{\mathrm {(}}{\theta }{\mathrm {)}}\) and the decision alternatives considered are collected in the vector \(\mathcal {A}\). According to the expected utility theory, the optimal decision alternatives a opt is the choice of obtaining the minimum expected cost:
$$ {}{a_{\text{opt}}} = \arg \mathop {\min }\limits_{a \in {\mathrm{{\mathcal{A}}}}} {{\mathop\mathrm{E}\nolimits}_{\Theta }}[C(a,{\theta })] = \arg \mathop {\min }\limits_{a \in {\mathrm{{\mathcal{A}}}}} \int\limits_{\Theta} {C(a,{\theta })} f({\theta }){\mathop\mathrm{d}\nolimits} {\theta } $$
(11)
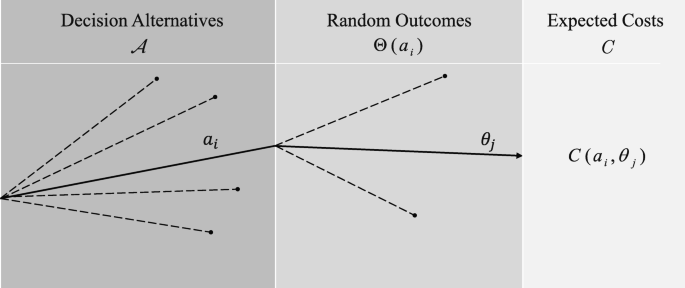
Decision tree for prior decision analysis
Full size image
where C(a,θ) is the cost function related to the structural state θ and the corresponding action a.
The posterior decision analysis corresponds to the scenario that additional information x has been achieved, on the basis of which the probabilistic models related to the decision context may be updated (Bayesian updating), see Fig. 4. The posterior decision analysis is in principle identical to the prior decision analysis, with the exception that the basis for the probabilistic modeling of uncertainty has been enhanced. The optimal posterior decision action \(a^{\prime }_{\text {opt}}\) can be made by:
$$ {}{a^{\prime}_{\text{opt}}} = \arg \mathop {\min }\limits_{a \in {\mathrm{{\mathcal{A}}}}} {{\mathop\mathrm{E}\nolimits}_{{\Theta^{\prime}}}}[C(a,{\theta |x})] = \arg \mathop {\min }\limits_{a \in {\mathrm{{\mathcal{A}}}}} \int\limits_{{\Theta^{\prime}}} {C(a,{\theta })} f({\theta }{|}x){\mathop\mathrm{d}\nolimits} {\theta } $$
(12)
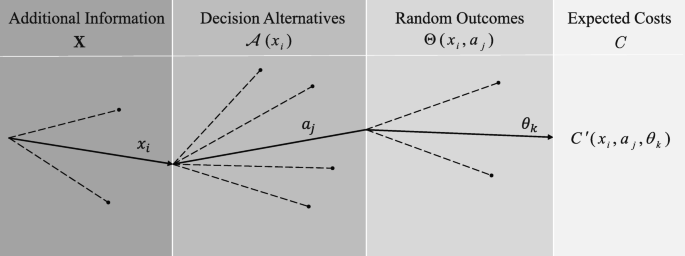
Decision tree for posterior decision analysis
Full size image
it should be noted that the definition of the set of decision alternatives, \(\mathcal {A}\), here is manifold. In Raiffa and Schlaifer [10], decision alternatives are simply collected in the vector \(\mathcal {A}\). It is up to the user/reader of the decision analytical framework to interpret this vector. This essentially means that the vector \(\mathcal {A}\) may contain several different classes of decision alternatives which may or may not be invoked depending on the information which is collected in the future. Such classes of decision alternatives might include exploratory decisions (decisions on different means to collect information) such as inspections, monitoring, proof load testing, model tests, specimen material tests, observations of load and degradation processes together with review of design basis/ as built drawings and manufacturing protocols. Another class of decision alternatives of course concerns changing the physics (repairs/strengthening/change of use, etc.). The details of this for the VoI analysis will be discussed in the next section.
The benefits of additional information x can be correspondingly quantified by the difference between the expected costs under the posterior and prior optimal actions, which is defined as conditional value of information (CVI):
$$ \begin{array}{l} {\text{CVI}}(x) = {{\mathrm{E}}_{\Theta^{\prime}}}[C({a_{{\text{opt}}}},\theta |x)] - {{\mathrm{E}}_{\Theta^{\prime}}}[C({{a^{\prime}}_{{\text{opt}}}},\theta |x)]\\ = \int\limits_{\Theta^{\prime}} {[C({a_{{\text{opt}}}},\theta |x)} - C({{a^{\prime}}_{{\text{opt}}}},\theta |x)]f(\theta |x){\mathrm{d}}\theta \end{array} $$
(13)
It can seen from Eq. 13 that the \(a^{\prime }_{\text {opt}}\) will bring more benefits than the a opt, meaning that CVI is a non-negative value.
Generally, obtaining additional information is associated with cost. Optimizing decisions with respect to collection of additional information is thus an effective means to help improve decisions on how to collect information before actually acquiring it. The key for this scenario is to consider all possible outcomes from the measurements on the basis of given probabilistic model of the outcomes, as shown in Fig. 5. Therefore, the collection of all the possible additional information may be modeled as a vector of random variables X with a designated (possibly subjective) probability distribution. In this case, the potential benefits associated with collecting the additional information are referred to as the expected value of information (EVI), which can be calculated by:
$$ {\text{EVI}} = {{\mathrm{E}}_{\mathbf{X}}}[{\text{CVI}} (x)] $$
(14)
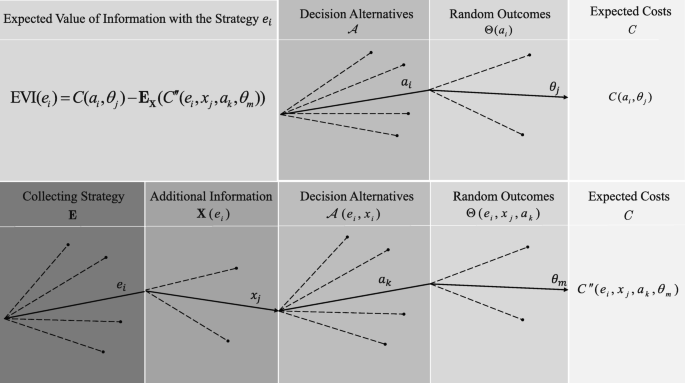
Decision tree for preposterior decision analysis
Full size image
As a result of CVI(x)≥0, the EVI in the framework of Bayesian pre-posterior decision analysis cannot be negative, which means that the additional information can always bring positive benefits without the consideration of itself costs.
Modelling aspects in value of information analysis
In this section, modelling methods accounting for the information representation, probabilistic model and utility function, together with the discussion of limited modelling aspects in the VoI analysis process, are described and discussed in relation to the relevant existing research, respectively.
Information modelling
The question of how to model information in an appropriate way is a crucial one in the VoI analysis, and two cases of information types are proposed [10]: perfect information, x p, and imperfect information, x ip. The distinction between the two types of information concerns the uncertainty associated with the information itself. Perfect information, as the term suggests, can directly reflect the true state of the variable, θ, without any uncertainty; this would correspond to an ideal situation, but is still a useful consideration as will be discussed later. Correspondingly, imperfect information refers to the case where the additional information related to the state of target variable, θ, is either indirect or associated with random noise, such as is generally the case in real world experimentation, sampling, and so on. The case of imperfect information is also referred to as sample information in Raiffa and Schlaifer [10]. While it is true that the decision maker usually cannot obtain perfect information, idealized consideration of the case of the expected value for perfect information (EVPI) is nevertheless of great practical interest. This is due to the fact that the computational efforts associated with EVPI analysis are far less than those required for the case of imperfect information and the fact that the value of imperfect information cannot exceed the value of perfect information. The EVPI case is thus a very useful first step in assessing whether collection of information at all has the potential to exceed the costs of collecting the information. If this proves to be the case, more detailed VoI analysis can be performed on the imperfect information.
The consideration of uncertainties associated with the relevant information in civil and infrastructure engineering is an essential issue in engineering risk analysis. This e.g. concerns the probability of detection (PoD) for inspection techniques [54], model uncertainties associuated with SHM information [30], among others. With respect to measurement errors, there are two types of error models that have been widely adopted: the additive error model and the multiplicative error model [55]. The additive error model is defined as:
$$ {{x}} = h_{{add}}(\mathbf{\theta }) + \mathbf{\varepsilon } $$
(15)
where x is the information, ε is defined as the generalized error, including the measurement errors associated with the selected measurement technique, the uncertainty associated with the functional relationship between the measurements and the actual state (also referred to as condition indicators), etc. h add is used to represent the relationship between the information and target variable in the additive error model. Whereas the multiplicative error model is defined as:
$$ {{x}} = h_{{mul}}(\mathbf{\theta }) e^{\mathbf{\varepsilon }} $$
(16)
where h mul (θ) is used to represent the relationship between the information and target variable in the multiplicative error model.
Two types of error were suggested to take into account in Feltham [12]: variability and bias. Variability refers to random noise affecting the measurement process. This is often modeled as a zero-mean normal distribution. The variability cannot affect possible changes in the expected value of the estimated results obtained from the data, whereas bias refers to the difference between the expected value of the measurement information and the actual state due to possible systematic errors (non-ergodic variations) in the measurement method. When a measurement can be regarded as an unbiased experiment, the zero-mean normal distribution could be employed to model the random error of the measurement, which is mainly used to discuss the influence of the error of information in existing studies [23, 27, 56, 57]. Whereas the measurement system will inevitably have systematic errors, to this end, the significance of biases associated with inspection results is investigated in Ali et al. [58], furthermore, the often prevailing dependency of inspection performance over consecutive inspections are also discussed. In addition, the property of imperfect information can also be modeled as the likelihood function of the performance indicator, see e.g. [59, 60].
Recently, an in-depth discussion on the representation of information in decision analysis is conducted in Nielsen et al. [61], in which the information is categorized into five types according to the relationship between information and decisions:
-
The information is relevant and precise
-
The information is relevant but imprecise
-
The information is irrelevant
-
The information is relevant but incorrect
-
The flow of information is disrupted or delayed
Understanding and modelling the information source and corresponding flow is important and significantly affects the decision making and of course also the outcome of VoI analysis. To this end, a framework and the related processing methods of various types of information for eliminating the adverse consequence by misunderstanding the information are proposed. Moreover, structural life-cycle integrity management is a time-dependent decision-making process, in which the possible time-dependent properties of information and its modelling play important roles. The temporal modelling method of SHM information during the operational phase of structures is discussed and proposed in Zhang et al. [5].
Probabilistic modelling
In this subsection, probabilistic modelling is addressed in terms of two types: information source and likelihood function. Probabilistic modelling of the information source is the fundamental step in the decision process. As stated before, the probabilistic model of information in the prior decision analysis is regarded as the information source in the pre-posterior decision analysis [10]. In such a case, an a-priori available database related to the information and the corresponding probabilistic model is necessary, which means the benefit of additional information cannot be assessed through one or a few realizations of information. In addition, the accuracy of the model of the information source will significantly affect the correctness of the VoI analysis results, and the information may come from various sources, some of which may not be related to the decision scenario. When performing decision analysis, the source of the information may be unknown and may often come from possible competing or alternative systems [61]. As such, all relevant possible sources must be accounted for in modelling the probabilistic representation for a more adequate and accurate prior probabilistic information model. To this end, a novel big data technique based approach is presented as a means of developing probabilistic representations which makes full use of the prior available knowledge and the information collected through observations [62].
In Bayesian analysis, the information gained from new data is represented by the likelihood function, which plays a key role in Bayesian inference, the likelihood function of a hypothesis (H) given some data (D) is the probability of obtaining D given that H is true can be expressed as:
$$ L(h) = P(D|H = h) $$
(17)
The likelihood function is used to update the prior distribution to a posterior distribution through a well-known conditional probability theorem:
$$ P(\theta |D) \propto P(\theta) \times P(D|\theta) $$
(18)
where θ is the parameter of interest, P(θ) is the prior distribution of θ. A tutorial of likelihood function and its applications can be found in [63]. In civil and infrastructure engineering, there are three common ways to model the likelihood function for different purposes. The first way is to establish the likelihood function L(θ) through the relationship between the measurement outcome x and the corresponding mathematical function of actual state for the variable of interest θ with the consideration of additive measurement error ε (see Eq.15):
$$ L(\theta) = {f_{\varepsilon} }(x - h(\theta)) $$
(19)
where f ε (.) is the probabilistic model of the measurement error, this case often can be found in the value of imperfect information analysis [5, 23, 27, 57]. The second method is to directly establish the likelihood function by using existing experiments, some phenomenological of physics based models (e.g. finite element models) or past observations, such as probability of detection (PoD) for inspection technique, see e.g. [54, 59, 60]. Another way is to take the statistical uncertainty and the model uncertainty associated with a variable into account by introduction of so-called hyper-parameters. Then based on Bayes Theorem the posterior distribution of the random variable is obtained to reduce the relevant uncertainties. This way can be used in the decision scenarios related to the life-cycle management or decision making with respect to information collection in the context of spatial variability [5, 64, 65].
Utility modelling
The notion of utility is derived from the economic theory of value to describe the satisfaction a person obtains through consumption or use of a certain commodity. For investment, it refers to the satisfaction that investors obtain from different investment portfolios. Compared with the expected monetary value, when the utility is used as a decision criterion, it is usually necessary to consider the decision maker's attitude towards risk and the influence of psychological factors. The utility function is a representation to define individual preferences for goods or services beyond the explicit monetary value of those goods or services, which are often expressed as U(x1,x2,x3,...) which means that U, our utility, is a function of the quantities of x1,x2, and so on. In most existing publications of risk management of civil and infrastructure engineering, it is often assumed that the decision-maker is ideally rational and risk-neutral, see.e.g. [66], in this case, the utility function U can be written in:
$$ U(c(a,\theta)) = - c(a,\theta) $$
(20)
where c is a expected monetary value conditional on the action a and the state of system θ.
A number of studies have considered risk attitudes in engineering decisions, in Wang et al. [67], the utility functions relating to owners' financial capacity and risk-averse psychology are used to the pre-posterior decision analysis for optimal bridge inspection planning. In the decision making process, when the utility functions are consistent, it has been stated earlier and also shown in [27, 57] that VoI cannot be negative. Recently, it has been shown that VoI can be negative when the utility functions are different, due to the fact that different people have different risk attitudes at different stages of the decision process [68, 69].
Another situation is where the benefits of information cannot be measured in economic terms. In this case, quantitative VoI returns to its original purpose of the application, namely the value of its contribution to reducing uncertainty [8]. To a certain extent, VoI analysis can also be regarded as a special sensitivity analysis, which is used to obtain the optimum choice of information to reduce uncertainty in the system. For instance, the information entropy was utilized to optimize the location of sensors in the structure [70], a new formulation of information benefits, which takes rigorously into account both information gain and cost-related benefits, is employed in VoI analysis to optimize the sensor configuration of ultrasonic guided-wave inspection [71]. Moreover, the measure of VoI is not limited to the technical level, in the field of civil and infrastructure engineering, more attentions could be paid to the impact of information on the levels of the considered system and the society, including considerations on robustness and resilience in the representation of the utility function [61, 72].
Further VoI modelling aspects
Generally, in existing investigations of the VoI analysis, the collections of decision alternatives are the same in both scenarios, and the complete additional information of interest has been collected as well when making a decision. As stated earlier, in some cases, the additional information may not only help reduce the epistemic uncertainty of decision-making within the original decision alternatives, but also help decision-makers to expand the space of decision alternatives or changing the rule of decision making to optimize benefits. For instance, proof load information may allow for exploring further action alternatives [73], the inspection information may help the time-based maintenance transfer to the condition-based maintenance [74], among others [58, 75, 76]. To highlight this feature, the VoI analysis with extended decision alternatives is defined as the expected value of sample information and actions (EVSIA) in [73, 76], since it is computed as the difference of expected benefits from the prior decision and the optimal decision and action combination with the consideration of additional information. The forms of this extended VoI analysis can be represented by the decision tree shown in Fig. 6.
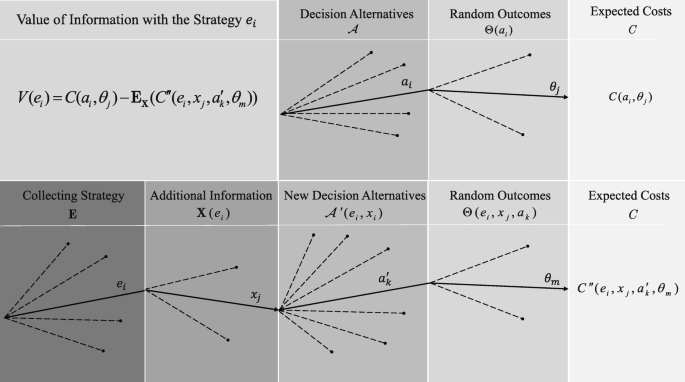
Decision tree for extended VoI analysis
Full size image
Other aspects
As mentioned earlier, without considering the cost of collecting information, the value of information from the Bayesian pre-posterior framework is strictly non-negative. This means that the additional relevant information will always bring benefits. The above conclusions may be contrary to people's perceptions because there is always some incorrect or fake information in reality [61]. The main reason lies in the fact that in Bayesian pre-posterior analysis, it is assumed that the probabilistic model of information accuracy has been known in advance, even if the information is imperfect, people can integrate the error of the information into the decision by constructing a likelihood function, thus eliminating the negative effects caused by imperfect information. Of course, this assumption is questionable, once people have a wrong knowledge of the source of information, there is no doubt that the results of the VoI analysis are meaningless.
In some cases the complete information of interest cannot be fully collected when making decisions. This should be adequately addressed when formulating the decision scenarios, in which the information should be divided into different types according to the state at the time of the decision [5]. Furthermore, the optimum time of decision making may be missed due to the time delay in the process of information processing and thereby leading to aggravated losses [12]. This point is worth noting since the amount of information collected from measurements on engineering structures might be rather substantial, and it might be practically infeasible to process the information in real-time. The question on how often to process collected information is central and so is the effect of possible delays associated with information processing.
VoI analysis in the field of civil and infrastructure engineering
This section provides a review of applied VoI analysis in the field of civil and infrastructure engineering. The section is divided into three main areas: computation methods, sensor arrangement optimization and engineering risk management. As the most important aspect, the last one will be divided into three items for further detailing. A summary of the reviewed literature below is listed in Table 1 according to the above divisions. In addition, the trend in the number of papers in this review, shown in Fig. 7, demonstrates that interest in VoI analysis has gained grown substantially over time.
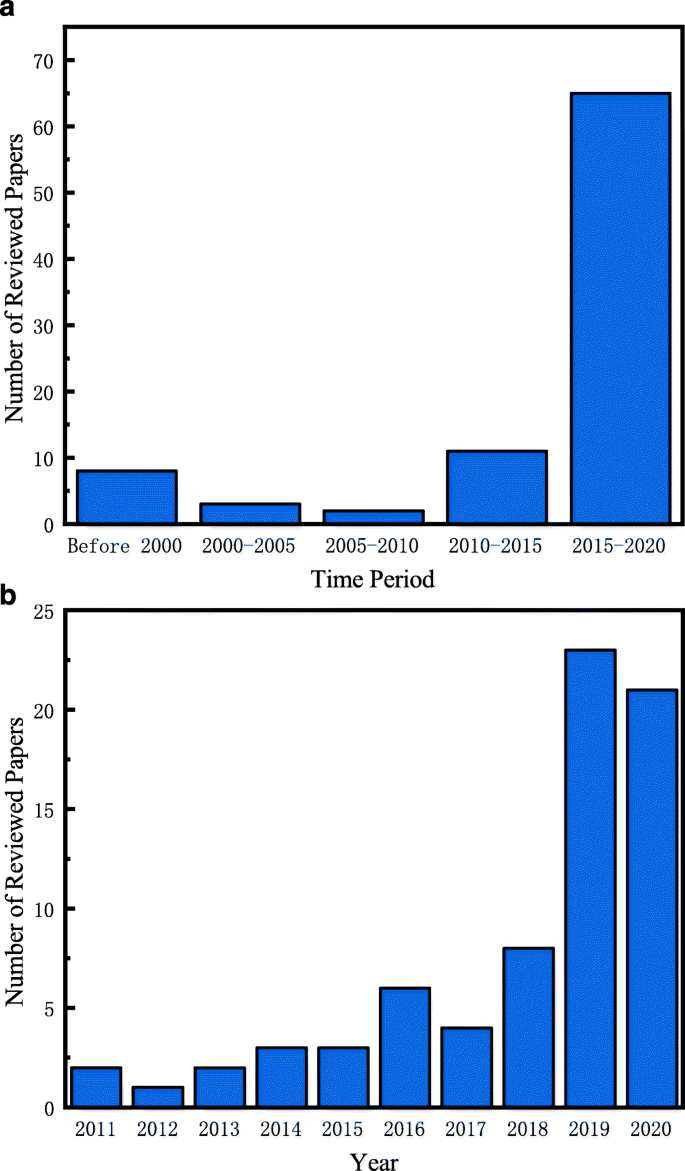
Trends in the number of published journal papers and conference papers in the present review. a Ten years period trend; b Annual trend from 2010 to 2020
Full size image
Full size table
Computation methods and efficient algorithm
The computation procedure of the VoI analysis can be divided into three steps: prior, posterior and pre-posterior decision analysis, which have been stated in detail earlier. The comprehensive computation framework of the VoI analysis can be found in [23, 27, 57]. Due to the nature of pre-posterior decision analysis, analysing VoI may require a significant computational effort, particularly in the field of civil and infrastructure engineering, where the probability of structural failure events is relatively small. In some extreme cases, the computational cost of the tested VoI is also higher than the cost of the test itself [23]. To this end, some efficient computation algorithms have been proposed based on the advanced surrogate model or graphical probabilistic models, see e.g. [77–79]. Further, machine learning algorithms may also be applied in support of VoI analysis, Vega and Todd [80] use a variational Bayesian neural network (BNN) to use SHM data as a means for updating the surrogate model, then by comparing the expected costs between the optimal decisions using inspection data only and the optimal decisions using a BNN surrogate model and the relevant SHM information to obtain the value of SHM information.
In the field of civil and infrastructure engineering, Straub [27] demonstrates how structural reliability methods could be used to effectively model the VoI and proposed an efficient algorithm for its computation based on the importance sampling method. In Straub et al. [29], the influence diagram is utilized to structure the VoI analysis, which provides a concise overview on the uncertain conditions and decisions scenarios. Some analysis methods for decision trees could also support the VoI analysis, in Thöns [30], the Bayesian extensive form of pre-posterior decision analysis, see [10] is used to reduce the computational cost of the pre-posterior decision analysis on the quantification of value of SHM information in the context of structural integrity and risk management. In this way, the VoI in dependency of structural system characteristics is quantified for a fixed SHM strategy. A similar analysis approach, which is called backward induction, is employed to develop the optimal decision-making for SHM systems in Cappello et al. [81]. The life-cycle management of engineering structures and infrastructures can be modeled as a sequential decision making problem to address the property of time dependent. In this case, VoI needs to be quantified in sequential decision-making scenarios, some efficient computation methods on the VoI have been developed based on MDP, POMDP, among others (see e.g. [50, 51, 82]). In addition to the sequential decision making, a holistic decision making approach is proposed to compute the VoI of periodic testing in Zou et al. [83]. To apply the VoI analysis easier, Agusta et al. [84] develop a software tool for moderately complex problems and demonstrated its progression in complexity and approaches to cope with computational challenges.
Sensor arrangement strategy optimization
Making optimal strategies for sensor arrangement is essentially a decision problem under uncertainty, which means that the expected utility theory can be used to provide a metric for assessing the quality of the design. The key, in this case, is the modeling of the utility function. Some efforts in this regard have been employed using information entropy based approaches to establish the utility function, see e.g. [70]. In Li and Der Kiureghian [85], three utility functions associated with quadratic loss, Shannon information and K-L divergence together with the elicitation of prior information are employed to make the prior decision on robust optimal sensor placement strategy. VoI analysis was not taken into account in their work due to the considered decision scenario. The similar research can be found in Capellari et al. [86], they perform the cost-benefit optimization of a sensor network by defining the density, type, and positioning of the sensors to be deployed with the Shannon information gain, and use the K-L divergence as the decision metric to optimize the sensor network. Malings and Pozzi [56] utilize the VoI as a metric to guide information collection strategy and optimal sensor placement in spatially distributed systems, they also discuss the computational complexity, the main limitation in pre-posterior decision analysis, and use some approximate techniques associated with the utility function and algorithm to decrease the computational cost. Thereafter, they extend their work from the static spatial system to the spatio-temporal systems [87]. Furthermore, Malings and Pozzi [88] find, in some cases, the VoI metric in the perspective of MDP lacks the property of submodularity, which will cause suboptimal solutions for sensing. To this end, they investigate the potential heuristic approach to avoid some of the shortcomings. In Cantero-Chinchilla et al. [71], they define utility function as the product of a normalized inverse cost function and the information gain, thereby the VoI will consist of two parts: the benefits of the expected information gain and the relative cost of implementation. It is somehow different from the VoI analysis in the economic field, in which the cost of obtaining information is not taken into account. More works of relevance can also be found in [89–93].
Engineering risk management
Modelling of relevant structural health information (SHI)
As reported in [94], the SHI type could be classified according to its characteristics in temporal and spatial scales together with the relationship between measurements and corresponding structural performance, see Table 2.
Full size table
There are two outcomes of an inspection action: no indication and an indication of damage. The merits of nondestructive inspection methods have been appreciated in the application of SIM [64, 95, 96]. Inspections may be used as a tool to reduce the uncertainty in the predicted deterioration and/or as a means of identifying deterioration before it becomes critical [95]. Straub and Faber [97] use VoI analysis to determine the optimal number of components to be inspected, thereby making the optimum RBI planning for the dependent system. Analogous to inspection, the Damage Detection System (DDS) also has the same two outcomes, the difference is that DDS can be modeled with a multivariate probability of indication based on the damage indicator value distribution for each damage state [98]. August et al. [99] combine SHM, DDS and inspection into the SIM and quantified the value of these SHI, a detailed investigation on the value of DDS can be found in Long et al. [60].
Proof load testing (PLT) is used to assess the actual load carrying capacity of a bridge, a successful proof loading test can immediately show that the resistance of the bridge is greater than the proof load, thereby reducing uncertainty in the bridge resistance and increasing the bridge reliability [100]. Nishijima and Faber [101] utilize the pre-posterior decision analysis to determine whether the PLT is beneficial and also to optimize the relevant parameters. A decision theoretic approach is utilized to demonstrate the optimal strategy for PLT procedures and collection of information in Kapoor et al. [102] and Schmidt et al. [103]. Further, on the basis of the PLT information, Brüske and Thöns [73] introduce a new concept, value of information and action (VoIA), to accommodate new decision scenarios to better quantify their VoI.
A SHM system may be viewed as a full-scale information collection system, which collects information of relevance for integrity management of structures [1]. A characteristic of the SHM system is that the types of information collected are diverse and continuous in time. In Pozzi and Der Kiureghian [23], VoI analysis is introduced and discussed in the context of SHM. Faber and Thöns [24, 104] propose a framework for the quantification of VoI and provide an outline on how to apply the framework in support of different types of decision contexts related to the design and life-cycle integrity management of structures. In Zhang et al. [5], a decision framework for SHM system arrangement is proposed to maximize the expected net gain from the implementation of the SHM system based on the VoI analysis.
The SHI analyses can be conducted throughout the life cycle phase accounting for the intact, hazard, constituent, and system damage and failure states (see Fig. 8), the details of relevance may be found in Thöns [94]. In the following, a review of existing research will be given in two main service life phases.
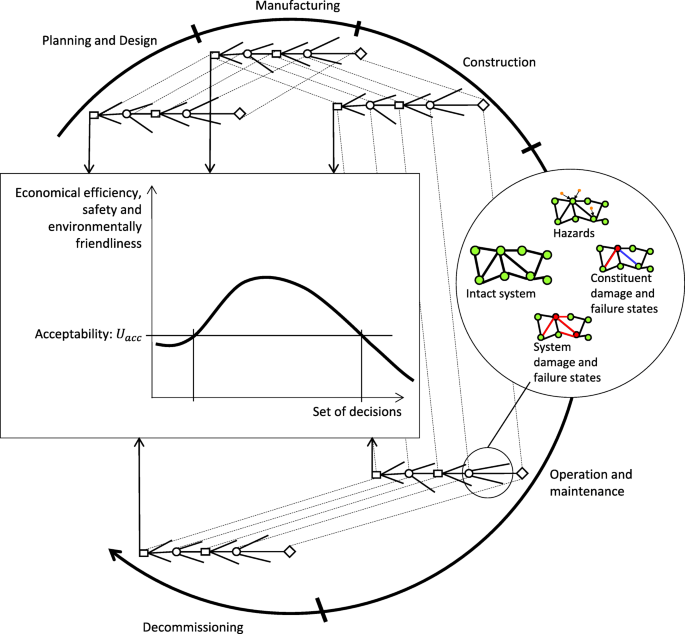
Illustration of a value of SHI analyses throughout the life cycle phase [94]
Full size image
Planning and design phase
In principle, structural design is a decision-making problem, and its purpose is to determine the structural design that maximizes the expected utility of the corresponding decision maker [105]. Before realizing a design, the available knowledge is limited and any prediction of future performance is associated with large uncertainties, the epistemic uncertainty can be decreased by additional experiment or exploration information of relevance. In this case, VoI analysis can provide support for the code and standard calibration, the structural prototype development and design by testing, together with the decision-making of information collection strategies during the design phase [94]. Some illustrative examples associated with the utilization of the VoI analysis in the design phase can be found in [17, 53]. In Espinosa and Köhler [106, 107], to efficiently allocate the resources at the design point in time of the offshore wind turbine support structure, the VoI analysis is utilized to investigate the potential benefits of site-specific information on avoiding the resonance. Brüske and Thöns [73] study the influence of additional information on structural design, and quantify its potential benefits on reducing the LCC. They further utilize the case of proof load testing to demonstrate the influence of the corresponding information on the selection of wind turbine substructure types, during the design phase.
Operation and maintenance phase
Information gathered by SHI could substantially contribute to providing more knowledge on the state of structures including their performance, loading and environmental conditions, which is beneficial to the prediction of the structural future performance and the planning of operation and maintenance. The main part of the existing works on the VoI analysis is focused on this aspect, which may be partitioned according to the following classification:
-
Optimal operation and maintenance (O &M) and structural integrity management (SIM) strategies
-
Management of environmental risks and emergencies
-
Assessment of system functionality
(1) Optimal operation and maintenance (O &M) and structural integrity management (SIM) strategies
In the field of O &M and SIM strategies, Madanat [21] applies the concept of VoI in infrastructure O &M decision based on hidden Markov decision processes and studies the impact of the uncertainty associated with non-destructive testing technology (NDT). An application framework of the concept of VoI in the SIM can be found in the work of Straub and Faber [22], providing an extension of generic RBI planning to optimize the inspection planning on the system-level based on VoI analysis. In the past twenty years, SHM systems have been generally appreciated in the context of O &M strategies, thus the quantification of the value of SHM information together with the VoI-based O &M strategy has recently been developed [23, 104].
Optimizing the operational strategies before implementing measurement systems and maintenance measures through Bayesian pre-posterior decision analysis is one of the main aspects in existing studies. In Qin et al. [65], the optimization of the SHM operation period is addressed in detail, which is based on the concept of VoI; where VoI reflects the reduction of the statistical uncertainty of the prior probabilistic model through SHM information. Hereafter, Zhang et al. [108] extend this method from component level to system level with the consideration of dependencies. Further investigations on the decision framework of SHM systems operation strategies with the consideration of RBI planning may be found in [5]. Thöns et al. [109] address a method of quantifying the value of SHM information in the context of SIM for a Daniels system subjected to fatigue deterioration. Further, this method is utilized and extended for the integrity management of a generic wind park [30]. In addition to the above studies, a joint consideration of monitoring and inspection in VoI analysis can be further found in Agusta et al. [99], in which the joint quantification of the VoI associated with inspections, SHM and damage detection system (DDS) is addressed. Further, Agusta [110] proposes a framework for the planning of the structural integrity and risk management of offshore structures by utilizing the value of information and action (VoIA) analysis, described in "Further VoI modelling aspects" section, to better investigate the value of additional information in conjunction with the performance of actions. In Long et al. [111], a VoI analysis focusing on the quantification of the value of structural and environmental information on the integrity management of an offshore wind turbine structure is conducted to show the benefits of the wind speed information and fatigue loading information. The concept of VoI analysis also provides a new perspective on the optimization of the inspection planning through maximizing of utility. In Liu and Frangopol [112], an optimum inspection planning is proposed aiming to provide the highest information gain for fatigue damage assessment and risk mitigation, which was also based on the pre-posterior decision analysis. Investigating the potential gains on the reduction of epistemic uncertainties using measurements before opting for costly maintenance actions may be feasible. To this end Goulet et al. [113] optimizes the sequence of actions including capacity interventions, demand limitation, measurements, model refinement and increase risk acceptance according to the maximum expected utility theorem. In addition to the time-dependent relationship in the decision problem, the nature of the spatial correlation is also an important issue to be considered. In Vereecken [114], a pre-posterior analysis framework for spatially degraded concrete structures is developed to optimize inspection and maintenance planning, in which the VoI is regarded as a metric for evaluating the feasibility of the decision. More investigations associated with the O&M stratigies and SIM can also be found in [98,115–120].
As mentioned earlier, sequential decision-making involving multiple decisions is usually used to model the life-cycle management scenario. Recently, the VoI in sequential O &M decision analysis has received widespread attentions. In Straub [27], an approximate solution is proposed to assess the VoI in sequential decision scenario, which only considers the information at predefined time instances. This solution may underestimate the overall VoI, but for many applications the approximation may be reasonable. For performance degradation that can be modeled as discrete Markov process, efficient numerical algorithms for solving the MDP can be utilized. Memarzadeh and Pozzi [50] illustrates how to assess the value of inspection and monitoring in the POMDP from the component-level to system-level by allocation of components. Further, based on the framework proposed in [50], Li and Pozzi [121] investigate how the features of long-term SHM information and of the O &M process influence the VoI. Additional investigations on discussing the VoI analysis within POMDP may be found in [44,51]. Recently, an aperiodic sequential inspection and condition-based maintenance policy with a VoI metric are proposed in Fauriat and Zio [122]. In their work, the average cost per unit time is selected as the optimized object, the inspection is regarded as the source of additional information which can be utilized the VoI analysis to quantify its potential benefits and further to optimize the inspection time. Moreover, a holistic VoI analysis of multiple inspection times considering the combined effect of life maintenance interventions can be found in Zou et al. [83,116].
As stated earlier, with the implementation of SHM systems, the maintenance alternatives or new decision rules in the context of SIM could be enriched to further reduce the expected LCC. In this case, the value of the reduction of expected LCC could be regarded as the extended form of VoI. Nilsson and Bertling [123] carry out an analysis for the LCCs for two different scenarios with and without CMS implementation, to gain an understanding of whether a CMS is profitable for the wind turbine farm. A similar approach can also be found in Nielsen and Sørensen [124], Zou et al. [74] and Ali et al. [58], in which the VoIs are the difference of LCCs with and without additional information.
Decisions on service life extensions may also be supported by additional information. Thereby VoI analyses may be employed to optimize the information collection strategy. In Thöns et al. [125], an approach for the quantification of the VoI for determining whether to extend the service life of a wind park is proposed, which is based on a LCC analysis and the direct and indirect consequences of structural damages and failures. Three SHM strategies are investigated to highlight that VoI may be regarded a very useful metric in the process of planning and operating SHM systems for service life extension of wind parks.
(2) Management of environmental risks and emergencies
Environmental effects on structures may lead to safety issues related to human life, functional disruptions and economic consequences. At the same time, in some extreme conditions, such as icing events, floods and earthquakes, it may cause public safety emergencies. In Bayraktarli and Faber [126], a city level risk-based decision analysis for earthquake risks based on a Bayesian Network is proposed to consider the additional observed information benefits on the uncertainty reduction.
In order to reduce the risks and support the emergency management for extreme weather condition, specific types of monitoring systems can be used to monitor or support the prediction of environmental conditions. In Roldsgaard et al. [127], the value of early warning of icing events is investigated on the basis of the monitoring of environmental conditions and short-term forecasting. A Bayesian network is to model probabilistically related to the ice accretion on bridge cables, which facilitates updating of the ice occurrence probability with the monitoring data. Rastayesh et al. [128] address the application of VoI and VoIA analysis for the installation of heating systems in wind turbine blades to reduce the risks associated with human fatality, functional disruptions, and/or economic losses.
Bridges over waterways can be adversely affected by scouring erosion, which, in some extreme cases, can rapidly compromise foundation stiffness and result in unacceptable movements or even collapse. Giordano et al. [59] propose a framework of the VoI analysis for monitoring systems of scoured bridges in emergency management, two actions are considered in the emergency management: open the bridge and close the bridge, associated to direct consequences and indirect consequences. A similar framework investigating the value of SHM information is applied to the case of post-earthquake emergency management in Giordano et al. [129]. Klerk et al. [130] explore the potential benefits of pore pressure monitoring for earthen flood defences; they also consider the climate change effects of the future loads. A similar investigation about the VoI analysis for snow loads monitoring systems can be found in Diamantidis et al. [131].
Terrorist attacks on engineering structures can be regarded as deliberate human-made events with high consequences, including direct structural damage and loss of life, as well as indirect negative social effects. Thöns and Stewart [132] address the assessment framework of the cost efficiency of risk mitigation strategies for terrorist attacks with improvised explosive devices for an iconic bridge structure on the basis of pre-posterior decision analysis. Further, based on their previous assessment framework, Thöns and Stewart [133] provide decision support for identifying the potential benefits of various risk reducing strategies for large governmental buildings with the consideration of Life Quality Index (LQI).
(3) Assessment of system functionality
The development and management of the societal infrastructure is a central task for the continuous development of society. As such, the system functionalities like infrastructure robustness and resilience [134] can also be regarded as a reference for decision indicators. In Thöns et al. [125], three SHM strategies are proposed for the wind park operation associated with direct and indirect benefits and consequences. Thereby, the corresponding improvements in robustness and vulnerability can be evaluated, which provide a wider perspective of the VoI analysis. In Miraglia et al. [135], VoI analysis is utilized for quantifying the potential benefits of different monitoring strategies applied as a means for improving the resilience of an interconnected system comprised by infrastructure, economy and environment. Further, Qin et al. [136] illustrate the utilization of VoI analysis in the case of resilience modelling and management of wind turbine park. In the optimal decision-making process, they maximize the service life benefits under the constraint of a maximum acceptable annual probability of resilience failure. Subsequently, the VoI is quantified under this scenario; more details of relevance may also be found in Qin et al. [72].
Summary and research challenges
As an important and effective means of supporting engineering decisions in the context of optimal management of additional information, VoI analysis has achieved systematic progress in the field of civil and infrastructure engineering, and has been widely studied not only in operation and maintenance decisions involving economic factors, but also in the optimal design of structures and monitoring systems. The present work illustrates different origins of the VoI analysis formulations on the basis of information and communication theory and Bayesian decision theory, and also highlights the importance of awareness of the underlying assumptions and definitions, and coherence with the modeled systems and scenarios. Thereafter, a literature review is provided on available VoI related research in the field of civil and infrastructure engineering. This review reveals the growing interest in VoI analysis over the past decade with the rise of big data related technologies, in the hope of providing a systematic review for researchers to assist future research in this field. The concept of and the logics underlying VoI analysis provide a perspective based on the potential benefits and how additional information about the perception of engineering structures can be better used to assist decision makers in relevant engineering decisions.
However, there are still substantial challenges in how to efficiently calculate VoI and better conduct VoI analyses for the life-cycle integrity management of engineering structures, and the directions worthy of future research are very rich. Some summaries and outlooks on the VoI analysis in the field of civil and infrastructure engineering are illustrated in the following:
(1) Information modelling: At present, the modelling of information is still mainly based on the classification of information in classical Bayesian decision theory. However, as the behaviour of engineering structures is diverse and coupled, the decomposition and identification of information is important for the VoI analysis, and further refinement of information in engineering from the perfect and imperfect information into more realistic categories has been proposed in the existing literature. Similarly, modelling the spatio-temporal correlation and delay of information is also necessary, and not much attention has been paid to this aspect in existing studies.
(2) Probabilistic modelling: With the emerging technology involving big data and industry 4.0, information would require a rigorous probabilistic modeling and assessment of their value. The selection of a prior probabilistic representation of information and the construction of a likelihood function of information and reality is the most important aspects of VoI analysis. The accuracy of the prior model plays a decisive role for information value, as it determines the source of information in the pre-posterior model. At present, in many practical problems, there is information that can be captured without a more mature prior model, and there is not yet a more mature analytical framework for the VoI analysis in such cases.
(3) Utility modelling: The main utility criterion in the existing literature is still the expected monetary value, but it is more important for decision makers to consider the utility of stakeholder risk attitudes, while broad indicators (such as system functionality) should also be considered in the VoI analysis. How to develop reasonable utility rating criteria is also an important task in measuring the value of additional information. Such issues have been noted by scholars and are currently being studied in greater depth.
(4) Scenario modelling: Modelling decision scenarios and the way in which information is used in decision-making in a suitable manner is important for accurately measuring the value of additional information in the decision-making of engineering structures. As such, full life cycle modeling including the dependencies between the design, construction and operation phases should be developed. Especially in sequential or time-dependent decision scenarios, time series information may not be fully observable at some of these decision time points. In addition, the correct use of information is another issue that requires careful consideration, as inappropriate use of information in certain scenarios can have a negative impact.
Availability of data and materials
All data generated or analyzed during this study are included in this published article.
Declarations
Abbreviations
- VoI:
-
Value of information
- NDT:
-
Non-destructive testing
- PLT:
-
Proof load testing
- SHM:
-
Structural health monitoring
- RS:
-
Remote sensing
- DIKW:
-
Data-information-knowledge-wisdom
- EUT:
-
Expected utility theory
- MDP:
-
Markov decision process
- RBI:
-
Risk-based inspection
- K-L:
-
Kullback-Leibler divergence
- EVI:
-
Expected value of information
- ENGS:
-
Expected net gain of sampling
- POMDP:
-
Partially observable Markov decision process
- CVI:
-
Conditional value of information
- PoD:
-
Probability of detection
- EVSIA:
-
Expected value of sample information and actions
- BNN:
-
Bayesian neural network
- SHI:
-
Structural health information
- DDS:
-
Damage detection system
- O&M:
-
Operation and maintenance
- SIM:
-
Structural integrity management
- LCC:
-
Life-cycle cost
- LQI:
-
Life quality index
References
- 1
Ou J, Li H (2010) Structural health monitoring in mainland China: review and future trends. Struct Health Monit 9(3):219–231.
Article Google Scholar
- 2
Farrar CR, Worden K (2012) Structural Health Monitoring: A Machine Learning Perspective. https://doi.org/10.1002/9781118443118.
- 3
Thöns S, Limongelli M, Ivankovic AM, Faber M, Val D, Chryssanthoplous M, Lombaert G, Döhler M, Straub D, Chatzi E, et al (2017) Progress of the COST action TU1402 on the quantification of the value of structural health monitoring In: IWSHM-11th International Workshop on Structural Health Monitoring.
- 4
Bao Y, Chen Z, Wei S, Xu Y, Tang Z, Li H (2019) The state of the art of data science and engineering in structural health monitoring. Engineering 5(2):234–242.
Article Google Scholar
- 5
Zhang W-H, Qin J, Lu D-G, Thöns S, Faber MH (2020) VoI-informed decision-making for SHM system arrangement. Struct Health Monit. https://doi.org/10.1177/1475921720962736.
- 6
Nordhaus WD, Popp D (1997) What is the value of scientific knowledge? An application to global warming using the PRICE model. Energy J 18(1).
- 7
Shannon CE (1948) A mathematical theory of communication. Bell Syst Tech J 27(3):379–423.
MathSciNet MATH Article Google Scholar
- 8
McCarthy J (1956) Measures of the value of information. Proc Natl Acad Sci U S A 42(9):654.
MATH Article Google Scholar
- 9
Howard RA (1966) Information value theory. IEEE Trans Syst Sci Cybernet 2(1):22–26.
Article Google Scholar
- 10
Schlaifer R, Raiffa H (1961) Applied Statistical Decision Theory. Harvard University Press, Boston.
MATH Google Scholar
- 11
von Neumann J, Morgenstern O (2007) Theory of Games and Economic Behavior. https://doi.org/10.2307/3610940.
- 12
Feltham GA (1968) The value of information. Account Rev 43(4):684–696.
Google Scholar
- 13
Miller AC (1975) The value of sequential information. Manag Sci 22(1):1–11.
MATH Article Google Scholar
- 14
Yokota F, Thompson KM (2004) Value of information analysis in environmental health risk management decisions: past, present, and future. Risk Anal Int J 24(3):635–650.
Article Google Scholar
- 15
Bratvold RB, Bickel JE, Lohne HP, et al (2009) Value of information in the oil and gas industry: past, present, and future. SPE Reserv Eval Eng 12(04):630–638.
Article Google Scholar
- 16
Keisler JM, Collier ZA, Chu E, Sinatra N, Linkov I (2014) Value of information analysis: the state of application. Environ Syst Decis 34(1):3–23.
Article Google Scholar
- 17
Benjamin JR, Cornell CA (2014) Probability, Statistics, and Decision for Civil Engineers. McGraw-Hill, New York.
Google Scholar
- 18
Ang AHS, Tang WH (1975) Probability Concepts in Engineering Planning and Design: Decision, risk and reliability. Wiley.
- 19
Thoft-Christensen P, Sørensen J (1987) Optimal strategy for inspection and repair of structural systems. Civ Eng Syst 4(2):94–100.
Article Google Scholar
- 20
Fujita M, Schall G, Rackwitz R (1989) Adaptive reliability-based inspection strategies for structures subject to fatigue In: Structural Safety and Reliability, 1619–1626.. ASCE.
- 21
Madanat SM (1991) Optimizing sequential decisions under measurement and forecasting uncertainty: Application to infrastructure inspection, maintenance and rehabilitation. PhD thesis. Massachusetts Institute of Technology.
- 22
Straub D, Faber MH (2005) Risk based inspection planning for structural systems. Struct Saf 27(4):335–355.
Article Google Scholar
- 23
Pozzi M, Der Kiureghian A (2011) Assessing the value of information for long-term structural health monitoring In: Health Monitoring of Structural and Biological Systems 2011, 79842.. International Society for Optics and Photonics.
- 24
Faber MH, Thöns S (2014) On the value of structural health monitoring In: 22nd Annual Conference on European Safety and Reliability, 2535–2544.. CRC Press.
- 25
Faber MH (2017) Risk informed structural systems integrity management: a decision analytical perspective In: International Conference on Offshore Mechanics and Arctic Engineering, 009–12040.. American Society of Mechanical Engineers.
- 26
COST (2014) Memorandum of understanding: TU 1402. European Cooperation in the Field of Scientific and Technical Research Brussels.
- 27
Straub D (2014) Value of information analysis with structural reliability methods. Struct Saf 49:75–85.
Article Google Scholar
- 28
Zonta D, Glisic B, Adriaenssens S (2014) Value of information: impact of monitoring on decision-making. Struct Control Health Monit 21(7):1043–1056.
Article Google Scholar
- 29
Straub D, Chatzi E, Bismut E, Courage W, Döhler M, Faber MH, Köhler J, Lombaert G, Omenzetter P, Pozzi M, et al (2017) Value of information: A roadmap to quantifying the benefit of structural health monitoring In: ICOSSAR-12th International Conference on Structural Safety & Reliability.
- 30
Thöns S (2018) On the value of monitoring information for the structural integrity and risk management. Comput Aided Civ Infrastruct Eng 33(1):79–94.
Article Google Scholar
- 31
Steinberg DM, Hunter WG (1984) Experimental design: review and comment. Technometrics 26(2):71–97.
MathSciNet MATH Article Google Scholar
- 32
Paninski L (2005) Asymptotic theory of information-theoretic experimental design. Neural Comput 17(7):1480–1507.
MATH Article Google Scholar
- 33
Stratonovich RL (2020) Theory of Information and Its Value. Springer, Switzerland.
MATH Book Google Scholar
- 34
Kjærulff UB, Madsen AL (2013) Bayesian Networks and Influence Diagrams: A Guide to Construction and Analysis (Second Edition).
- 35
DeGroot MH (2005) Optimal Statistical Decisions, Vol. 82. John Wiley & Sons, New Jersey.
MATH Google Scholar
- 36
Kamiński B, Jakubczyk M, Szufel P (2018) A framework for sensitivity analysis of decision trees. CEJOR 26(1):135–159.
MathSciNet MATH Article Google Scholar
- 37
Pratt JW, Raiffa H, Schlaifer R, et al (1995) Introduction to Statistical Decision Theory. MIT press, Cambridge.
MATH Google Scholar
- 38
Straub D, Faber MH (2006) Computational aspects of risk-based inspection planning. Comput Aided Civ Infrastruct Eng 21(3):179–192.
Article Google Scholar
- 39
Alagoz O, Hsu H, Schaefer AJ, Roberts MS (2010) Markov decision processes: a tool for sequential decision making under uncertainty. Med Dec Making 30(4):474–483.
Article Google Scholar
- 40
Bellman R (1957) A Markovian decision process. J Math Mech 6(5):679–684.
MathSciNet MATH Google Scholar
- 41
Markov AA (1971) Extension of the limit theorems of probability theory to a sum of variables connected in a chain. Dyn Probabilistic Syst 1:552–577.
Google Scholar
- 42
Hu Q, Yue W (2007) Markov Decision Processes with Their Applications, Vol. 14. Springer, New York.
MATH Google Scholar
- 43
Åström KJ (1965) Optimal control of Markov processes with incomplete state information. J Math Anal Appl 10(1):174–205.
MathSciNet MATH Article Google Scholar
- 44
Papakonstantinou KG, Shinozuka M (2014) Planning structural inspection and maintenance policies via dynamic programming and Markov processes. Part II: POMDP implementation. Reliab Eng Syst Saf 130:214–224.
Article Google Scholar
- 45
Chatterjee K, Chmelik M, Tracol M (2016) What is decidable about partially observable Markov decision processes with ω-regular objectives. J Comput Syst Sci 82(5):878–911.
MathSciNet MATH Article Google Scholar
- 46
McMillan D, Ault G (2008) Condition monitoring benefit for onshore wind turbines: sensitivity to operational parameters. IET Renew Power Gener 2(1):60–72.
Article Google Scholar
- 47
Thöns S, McMillan D (2012) Condition monitoring benefit for offshore wind turbines In: 12th International Conference on Probabilistic Methods Applied to Power Systems.
- 48
Thöns S, McMillan D (2014) Condition monitoring benefit for operation support of offshore wind turbines In: Reliability Modeling and Analysis of Smart Power Systems, 169–182.. Springer, New Delhi.
Chapter Google Scholar
- 49
May A, McMillan D, Thöns S (2015) Economic analysis of condition monitoring systems for offshore wind turbine sub-systems. IET Renew Power Gener 9(8):900–907.
Article Google Scholar
- 50
Memarzadeh M, Pozzi M (2016) Value of information in sequential decision making: Component inspection, permanent monitoring and system-level scheduling. Reliab Eng Syst Saf 154:137–151.
Article Google Scholar
- 51
Andriotis C, Papakonstantinou K, Chatzi E (2019) Value of structural health monitoring quantification in partially observable stochastic environments. arXiv preprint arXiv:1912.12534.
- 52
Faber MH, Stewart MG (2003) Risk assessment for civil engineering facilities: critical overview and discussion. Reliab Eng Syst Saf 80(2):173–184.
Article Google Scholar
- 53
Faber MH (2012) Statistics and Probability Theory - In Pursuit of Engineering Decision Support. Springer, Netherlands.
MATH Google Scholar
- 54
Faber MH, Engelund S, Sørensen JD, Bloch A (2000) Simplified and generic risk based inspection planning In: Simplified and Generic Risk Based Inspection Planning.
- 55
Tian Y, Huffman GJ, Adler RF, Tang L, Sapiano M, Maggioni V, Wu H (2013) Modeling errors in daily precipitation measurements: Additive or multiplicative?Geophys Res Lett 40(10):2060–2065.
Article Google Scholar
- 56
Malings C, Pozzi M (2016) Value of information for spatially distributed systems: application to sensor placement. Reliab Eng Syst Saf 154:219–233.
Article Google Scholar
- 57
Konakli K, Sudret B, Faber MH (2016) Numerical investigations into the value of information in lifecycle analysis of structural systems. ASCE-ASME J Risk Uncertain Eng Syst, Part A: Civil Engineering 2(3):4015007.
Google Scholar
- 58
Ali K, Qin J, Faber MH (2020) On information modeling in structural integrity management. Struct Health Monit:1475921720968292.
- 59
Giordano PF, Prendergast LJ, Limongelli MP (2020) A framework for assessing the value of information for health monitoring of scoured bridges. J Civ Struct Health Monit:1–12.
- 60
Long L, Döhler M, Thöns S (2020) Determination of structural and damage detection system influencing parameters on the value of information. Struct Health Monit. https://doi.org/10.1177/1475921719900918.
- 61
Nielsen L, Tølbøll Glavind S, Qin J, Faber MH (2019) Faith and fakes–dealing with critical information in decision analysis. Civ Eng Environ Syst 36(1):32–54.
Article Google Scholar
- 62
Glavind ST, Sepulveda JG, Qin J, Faber MH (2019) Systems modeling using big data analysis techniques and evidence In: 2019 4th International Conference on System Reliability and Safety (ICSRS), 51–59.. IEEE.
- 63
Etz A (2018) Introduction to the concept of likelihood and its applications. Adv Methods Pract Psychol Sci 1(1):60–69.
Article Google Scholar
- 64
Qin J, Faber MH (2012) Risk management of large RC structures within spatial information system. Comput Aided Civ Infrastruct Eng 27(6):385–405.
Article Google Scholar
- 65
Qin J, Thöns S, Faber MH (2015) On the value of SHM in the context of service life integrity management In: 12th International Conference on Applications of Statistics and Probability in Civil Engineering, 8.
- 66
Maes MA, Faber MH (2007) Preferences, utility and risk perception in engineering decision making. Int J Risk Assess Manag 7(6-7):813–827.
Article Google Scholar
- 67
Wang J, Qin Q (2008) Optimum bridge inspection/repair arrangement based on time-dependent reliability and pre-posterior decision. Eng Mech 25(4):140–145.
Google Scholar
- 68
Bolognani D, Verzobio A, Tonelli D, Cappello C, Glisic B, Zonta D, Quigley J (2018) Quantifying the benefit of structural health monitoring: what if the manager is not the owner?Struct Health Monit 1:17.
Google Scholar
- 69
Bolognani D, Verzobio A, Zonta D, Quigley J (2019) Quantifying the benefit of SHM: can the VoI be negative? In: Sensors and Smart Structures Technologies for Civil, Mechanical, and Aerospace Systems 2019, 109701.. International Society for Optics and Photonics.
- 70
Papadimitriou C, Beck JL, Au S-K (2000) Entropy-based optimal sensor location for structural model updating. J Vib Control 6(5):781–800.
Article Google Scholar
- 71
Cantero-Chinchilla S, Chiachío J, Chiachío M, Chronopoulos D, Jones A (2020) Optimal sensor configuration for ultrasonic guided-wave inspection based on value of information. Mech Syst Signal Process 135:106377.
Article Google Scholar
- 72
Qin J, Faber MH (2019) Resilience informed integrity management of wind turbine parks. Energies 12(14):2729.
Article Google Scholar
- 73
Brüske H, Thöns S (2019) Value of pre-construction proof loading information for structural design. Wind Energy 22(12):1716–1732.
Article Google Scholar
- 74
Zou G, Banisoleiman K, González A, Faber MH (2019) Probabilistic investigations into the value of information: A comparison of condition-based and time-based maintenance strategies. Ocean Eng 188:106181.
Article Google Scholar
- 75
Nielsen JS, Tcherniak D, Ulriksen MD (2020) A case study on risk-based maintenance of wind turbine blades with structural health monitoring. Struct Infrastruct Eng:1–17.
- 76
Thöns S, Kapoor M (2019) Value of information and value of decisions In: 13th International Conference on Applications of Statistics and Probability in Civil Engineering.
- 77
Xu S (2003) Value of information in decision systems. PhD thesis. National University of Singapore.
- 78
Nielsen TD, Jensen FV (2009) Bayesian Networks and Decision Graphs. Springer, New York.
MATH Google Scholar
- 79
Chen Y, Javdani S, Karbasi A, Bagnell JA, Srinivasa SS, Krause A (2015) Submodular surrogates for value of information In: AAAI, 3511–3518.
- 80
Vega MA, Todd MD (2020) A variational Bayesian neural network for structural health monitoring and cost-informed decision-making in miter gates. Struct Health Monit:1475921720904543.
- 81
Cappello C, Zonta D, Glišić B (2016) Expected utility theory for monitoring-based decision-making. Proc IEEE 104(8):1647–1661.
Article Google Scholar
- 82
Srinivasan R, Parlikad AK (2013) Value of condition monitoring in infrastructure maintenance. Comput Ind Eng 66(2):233–241.
Article Google Scholar
- 83
Zou G, Faber MH, González A, Banisoleiman K (2021) Computing the value of information from periodic testing in holistic decision making under uncertainty. Reliab Eng Syst Saf 206:107242.
Article Google Scholar
- 84
Agusta A, Thöns S (2018) On the development of tools for decision analyses In: 1st International Conference on Structural Integrity for Offshore Energy Industry.
- 85
Li B, Der Kiureghian A (2016) Robust optimal sensor placement for operational modal analysis based on maximum expected utility. Mech Syst Signal Process 75:155–175.
Article Google Scholar
- 86
Capellari G, Chatzi E, Mariani S (2018) Cost–benefit optimization of structural health monitoring sensor networks. Sensors 18(7):2174.
Article Google Scholar
- 87
Malings C, Pozzi M (2018) Value-of-information in spatio-temporal systems: Sensor placement and scheduling. Reliab Eng Syst Saf 172:45–57.
Article Google Scholar
- 88
Malings C, Pozzi M (2019) Submodularity issues in value-of-information-based sensor placement. Reliab Eng Syst Saf 183:93–103.
Article Google Scholar
- 89
Krause A (2008) Optimizing sensing: theory and applications. PA: Carnegie Mellon University, Pittsburgh.
Google Scholar
- 90
Hoseyni SM, Di Maio F, Zio E (2019) VoI-based optimal sensors positioning and the sub-modularity issue In: 2019 4th International Conference on System Reliability and Safety (ICSRS), 148–152.. IEEE.
- 91
Malings C, Pozzi M (2016) Conditional entropy and value of information metrics for optimal sensing in infrastructure systems. Struct Saf 60:77–90.
Article Google Scholar
- 92
Yoshida I, Tasaki Y, Otake Y, Wu S (2018) Optimal sampling placement in a gaussian random field based on value of information. ASCE-ASME J Risk Uncertain Eng Syst, Part A: Civil Engineering 4(3):04018018.
Article Google Scholar
- 93
Melo C, Dann MR, Hugo RJ, Janeta A (2020) Optimal locations for non-destructive inspections to verify direct assessment of internally corroded pipelines. Upstream Oil Gas Technol 5:100008.
Article Google Scholar
- 94
Thöns S (2020) Quantifying the Value of Structural Health Information for Decision Support. https://www.jcss-lc.org/publicationsjcss/.
- 95
Faber MH (2002) Risk-based inspection: The framework. Struct Eng Int 12(3):186–195.
MathSciNet Google Scholar
- 96
Dong Y, Frangopol DM (2016) Incorporation of risk and updating in inspection of fatigue-sensitive details of ship structures. Int J Fatigue 82:676–688.
Article Google Scholar
- 97
Straub D, Faber MH (2004) System effects in generic risk-based inspection planning. J Offshore Mech Arct Eng 126(3):265–271.
Article Google Scholar
- 98
Thöns S, Döhler M, Long L (2018) On damage detection system information for structural systems. Struct Eng Int 28(3):255–268.
Article Google Scholar
- 99
Agusta A, Thöns S (2019) Value of information-based inspection, monitoring, and damage detection system planning In: 13th International Conference on Applications of Statistics and Probability in Civil Engineering.
- 100
Faber MH, Val DV, Stewart MG (2000) Proof load testing for bridge assessment and upgrading. Eng Struct 22(12):1677–1689.
Article Google Scholar
- 101
Nishijima K, Faber MH (2007) Bayesian approach to proof loading of quasi-identical multi-components structural systems. Civ Eng Environ Syst 24(2):111–121.
Article Google Scholar
- 102
Kapoor M, Schmidt JW, Sørensen JD, Thöns S (2019) A decision theoretic approach towards planning of proof load tests In: 13th International Conference on Applications of Statistics and Probability in Civil Engineering, 339.. Seoul National University.
- 103
Schmidt JW, Thöns S, Kapoor M, Christensen CO, Engelund S, Sørensen JD (2020) Challenges related to probabilistic decision analysis for bridge testing and reclassification. Diagn Proof Load Tests Bridges In: Front. Built Environ, 1–14. https://doi.org/10.3389/fbuil.2020.00014.
- 104
Thöns S, Faber MH (2013) Assessing the value of structural health monitoring In: 11th International Conference on Structural Safety and Reliability, 2543–2550.. CRC Press/Balkema.
- 105
Fischer K, Viljoen C, Köhler J, Faber MH (2019) Optimal and acceptable reliabilities for structural design. Struct Saf 76:149–161.
Article Google Scholar
- 106
Mendoza Espinosa J, Kohler J (2019) Risk-based design of an offshore wind turbine using VoI analysis In: 13th International Conference on Applications of Statistics and Probability in Civil Engineering (ICASP13).. Seoul National University Seoul, South Korea.
- 107
Espinosa JM, Köhler J (2019) Value of site-specific information for the design of offshore wind farms In: IABSE Symposium, Guimaraes 2019: Towards a Resilient Built Environment Risk and Asset Management - Report.
- 108
Zhang W, Lu D, Qin J, Faber MH (2019) Life cycle management of structural systems based on the optimal SHM strategy by VoI analysis In: 13th International Conference on Applications of Statistics and Probability in Civil Engineering, ICASP 2019International Conference on Applications of Statistics and Probability in Civil Engineering.. Seoul National University.
- 109
Thöns S, Schneider R, Faber MH (2015) Quantification of the value of structural health monitoring information for fatigue deteriorating structural systems In: 12th International Conference on Applications of Statistics and Probability in Civil Engineering.
- 110
Agusta A, Leira B, Thöns S (2020) Value of information-based risk and fatigue management for offshore structures. J Struct Integr Maint 5(2):127–141.
Google Scholar
- 111
Long L, Mai QA, Morato PG, Sørensen JD, Thöns S (2020) Information value-based optimization of structural and environmental monitoring for offshore wind turbines support structures. Renew Energy 159:1036–1046.
Article Google Scholar
- 112
Liu Y, Frangopol DM (2019) Utility and information analysis for optimum inspection of fatigue-sensitive structures. J Struct Eng 145(2):04018251.
Article Google Scholar
- 113
Goulet J-A, Der Kiureghian A, Li B (2015) Pre-posterior optimization of sequence of measurement and intervention actions under structural reliability constraint. Struct Saf 52:1–9.
Article Google Scholar
- 114
Vereecken E, Botte W, Lombaert G, Caspeele R (2020) Bayesian decision analysis for the optimization of inspection and repair of spatially degrading concrete structures. Eng Struct 220:111028.
Article Google Scholar
- 115
Skokandić D, Mandić Ivanković A (2020) Value of additional traffic data in the context of bridge service-life management. Struct Infrastruct Eng:1–20.
- 116
Zou G, Faber MH, González A, Banisoleiman K (2021) A simplified method for holistic value of information computation for informed structural integrity management under uncertainty. Mar Struct 76:102888.
Article Google Scholar
- 117
Agusta A, Faber M, Sørensen J, Schmidt J, Gardoni P, Omenzetter P, Thöns S (2020) Structural integrity and risk management based on value of information and action analysis. PhD thesis. Technical University of Denmark, Department of Civil Engineering.
- 118
van der Krogt MG, Klerk WJ, Kanning W, Schweckendiek T, Kok M (2020) Value of information of combinations of proof loading and pore pressure monitoring for flood defences. Struct Infrastruct Eng:1–16.
- 119
Long L, Alcover IF, Thöns S (2020) Utility-based analysis for optimal SHM durations and service life extension of welds on steel bridge deck. Struct Infrastruct Eng. https://doi.org/10.1080/15732479.2020.1866026.
- 120
Iannacone L, Francesco Giordano P, Gardoni P, Pina Limongelli M (2021) Quantifying the value of information from inspecting and monitoring engineering systems subject to gradual and shock deterioration. Struct Health Monit:1475921720981869.
- 121
Li S, Pozzi M (2019) What makes long-term monitoring convenient? a parametric analysis of value of information in infrastructure maintenance. Struct Control Health Monit 26(5):2329.
Article Google Scholar
- 122
Fauriat W, Zio E (2020) Optimization of an aperiodic sequential inspection and condition-based maintenance policy driven by value of information. Reliab Eng Syst Saf 204:107133.
Article Google Scholar
- 123
Nilsson J, Bertling L (2007) Maintenance management of wind power systems using condition monitoring systems–life cycle cost analysis for two case studies. IEEE Trans Energy Convers 22(1):223–229.
Article Google Scholar
- 124
Nielsen JS, Sørensen JD (2018) Computational framework for risk-based planning of inspections, maintenance and condition monitoring using discrete Bayesian networks. Struct Infrastruct Eng 14(8):1082–1094.
Article Google Scholar
- 125
Thöns S, Faber MH, Val DV (2017) On the value of structural health monitoring information for the operation of wind parks In: Proceedings of the Safety, Reliability, Risk, Resilience and Sustainability of Structures and Infrastructure, 12th International Conference on Structural Safety and Reliability, Wien Vienna, Austria, 6–10.
- 126
Bayraktarli YY, Faber MH (2011) Bayesian probabilistic network approach for managing earthquake risks of cities. Georisk 5:2–24.
Google Scholar
- 127
Roldsgaard JH, Georgakis CT, Faber MH (2015) On the value of forecasting in cable ice risk management. Struct Eng Int 25(1):61–70.
Article Google Scholar
- 128
Rastayesh S, Long L, Dalsgaard Sørensen J, Thöns S (2019) Risk assessment and value of action analysis for icing conditions of wind turbines close to highways. Energies 12(14):2653.
Article Google Scholar
- 129
Giordano PF, Miraglia S, Limongelli MG (2019) The benefit of permanent monitoring for seismic emergency management In: 9th International Conference on Structural Health Monitoring of Intelligent Infrastructure: Transferring Research Into Practice, SHMII 2019, 400–405.
- 130
Klerk WJ, Schweckendiek T, den Heijer F, Kok M (2019) Value of information of structural health monitoring in asset management of flood defences. Infrastructures 4(3):56.
Article Google Scholar
- 131
Diamantidis D, Sykora M (2019) Implementing information gained through structural health monitoring-proposal for standards In: 13th International Conference on Applications of Statistics and Probability in Civil Engineering, ICASP 2019International Conference on Applications of Statistics and Probability in Civil Engineering.. Seoul National University.
- 132
Thöns S, Stewart MG (2019) On decision optimality of terrorism risk mitigation measures for iconic bridges. Reliab Eng Syst Saf 188:574–583.
Article Google Scholar
- 133
Thöns S, Stewart MG (2020) On the cost-efficiency, significance and effectiveness of terrorism risk reduction strategies for buildings. Struct Saf 85:101957.
Article Google Scholar
- 134
Faber MH, Qin J, Miraglia S, Thöns S (2017) On the probabilistic characterization of robustness and resilience. Procedia Eng 198:1070–1083.
Article Google Scholar
- 135
Miragliaa S, Nielsen M, Thöns S, Stewart M (2017) Resilience of system by value of information and SHM In: Safety, Reliability, Risk, Resilience and Sustainability of Structures and Infrastructure, 2998–3007.. TU Verlag.
- 136
Qin J, Faber MH, Liu M, Zhang W, Lu D (2019) Value of information in resilience management of infrastructure systems In: IABSE Symposium 2019 Guimaraes: Towards a Resilient Built Environment-Risk and Asset Management. The Annual Symposium of the International Association for Bridge and Structural Engineering (IABSE) and the International Association for Shell and Spatial Structures (IASS), 1797–1807.. International Association for Bridge and Structural Engineering.
Download references
Acknowledgements
The first author is grateful to the China Scholarship Council (CSC) who supports his work at Aalborg University. The authors acknowledge financial support from the National Key Research and Development Plan of China (2018YFB2101001).
Funding
This work is supported by National Key Research and Development Plan of China (2018YFB2101001).
Ethics declarations
Competing interests
The authors declare that they have no competing interests.
Additional information
Publisher's Note
Springer Nature remains neutral with regard to jurisdictional claims in published maps and institutional affiliations.
Rights and permissions
Open Access This article is licensed under a Creative Commons Attribution 4.0 International License, which permits use, sharing, adaptation, distribution and reproduction in any medium or format, as long as you give appropriate credit to the original author(s) and the source, provide a link to the Creative Commons licence, and indicate if changes were made. The images or other third party material in this article are included in the article's Creative Commons licence, unless indicated otherwise in a credit line to the material. If material is not included in the article's Creative Commons licence and your intended use is not permitted by statutory regulation or exceeds the permitted use, you will need to obtain permission directly from the copyright holder. To view a copy of this licence, visit http://creativecommons.org/licenses/by/4.0/.
Reprints and Permissions
About this article
Cite this article
Zhang, WH., Lu, DG., Qin, J. et al. Value of information analysis in civil and infrastructure engineering: a review. J Infrastruct Preserv Resil 2, 16 (2021). https://doi.org/10.1186/s43065-021-00027-0
Download citation
-
Received:
-
Accepted:
-
Published:
-
DOI : https://doi.org/10.1186/s43065-021-00027-0
Keywords
- Value of information
- Bayesian decision theory
- Engineering risk analysis
- Optimum decision making
- Structural health information
How to Write a Systematic Review Civil Engineering
Source: https://jipr.springeropen.com/articles/10.1186/s43065-021-00027-0